Building Energy Boot Camp 2019 - Day 4
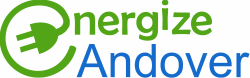
Today, we spent the entirety of the boot camp session working on the project we were assigned yesterday. I managed to finish the gallons per day (GPD) calculations and output the number of houses with GPDs higher than 300, 500, and 1000. I also wrote code to calculate the GPD of each street in Andover and write this, along with the GPD data for each house, into .csv files and Excel spreadsheets. However, as this code dealt with such a vast amount of data, I quickly noticed its long run time. Thus, I spent much of the work time optimizing my code. First, I made sure to drop any and all unnecessary columns, to greatly increase the total amount of cells in the pandas DataFrame. I also discovered cython, a tool that compiles Python code into C code, which runs many times faster than Python. Using cython, I managed to greatly decrease run times, but was still unable to bring them under 80 seconds. I have included the code and its output in the Code and Output section.
Images
Code and Output
import os
import time
import sqlalchemy
import numpy as np
import pandas as pd
import datetime as dt
# Used to record elapsed time
start_time = time.time()
# Define unit constants
CUBE_IN_PER_GALLON = 231
CUBE_IN_PER_CUBIC_FT = 1728
# The default length of time between 'prior_date' and 'current_date' if incomplete data is given
DEFAULT_DAYS_BETWEEN = 90
# The path to the sqlite file
db_path = os.path.join('SQLite', 'student.sqlite')
engine = sqlalchemy.create_engine('sqlite:///' + db_path) # Read the sqlite file into sqlalchemy
# Read the Water table into a dataframe
df_water = pd.read_sql_table('Water', engine, parse_dates=True)
# Delete unnecessary columns
df_water = df_water.drop(
columns=['service_id', 'account_number', 'service', 'meter_number', 'transaction_date', 'transaction_type', 'units',
'description', 'id'])
# Convert the 'current_reading' column into floats
df_water['current_reading'] = df_water['current_reading'].astype(float)
# Delete rows with 0 as a value for 'current_reading'
df_water = df_water[df_water['current_reading'] > 0]
# Convert the 'prior_date' and 'current_date' into datetime or None
def convert_to_datetime(s):
if s is None:
return None
try:
return dt.datetime.strptime(s, '%Y-%m-%d %H:%M:%S')
except ValueError:
return None
df_water['prior_date'] = df_water['prior_date'].apply(convert_to_datetime)
df_water['current_date'] = df_water['current_date'].apply(convert_to_datetime)
# Create an empty column, filled with 0s by default, to store gpd for the time period
# Data will be added to the column as it is calculated
gpd_list = [0 for counter in range(len(df_water.index))]
df_water['GPD'] = gpd_list
# Converts a numpy.datetime64 to a datetime.datetime object by converting dt64 to UTC time (for later use)
def datetime64_to_datetime(dt64):
return dt.datetime.utcfromtimestamp((dt64 - np.datetime64('1970-01-01T00:00:00Z')) / np.timedelta64(1, 's'))
# Convert values from cubic ft to gallons
df_water['current_reading'] = df_water['current_reading'].apply(lambda x: x * CUBE_IN_PER_CUBIC_FT / CUBE_IN_PER_GALLON)
# Create a dataframe to store the average GPD for each house, but leave it blank
df_base_dictionary = {'address_street_number': [], 'address_street_name': [], 'GPD': []}
df_gpd = pd.DataFrame(df_base_dictionary)
# Mark the 'GPD' column as floats
df_gpd['GPD'] = df_gpd['GPD'].astype(float)
print('Building table of average gallons used per day (average GPD)...')
# Iterate through every unique street
street_names = df_water['address_street_name'].unique()
for street in street_names:
# Get the list of all house numbers on that street
house_numbers = df_water[df_water['address_street_name'] == street]['address_street_number'].unique()
# Create a row for each house
for house in house_numbers:
df_gpd.loc[len(df_gpd)] = [house, street, 0]
print("Done!\n")
print("Calculating all average GPDs...")
# Go through every house and calculate the GPD
for index, row in df_gpd.iterrows():
# Grab the portion of the df_water that has to do with this house
df = df_water[df_water['address_street_name'] == row['address_street_name']]
df = df[df['address_street_number'] == row['address_street_number']]
df = df.sort_values(by='prior_date')
df = df.reset_index(drop=True)
for indx in range(1, len(df.index) - 1):
df = df.drop(index=indx) # Drop all but the first and last rows
df = df.reset_index(drop=True)
difference_in_gallons = df.iloc[1, :]['current_reading'] - df.iloc[0, :]['current_reading']
start_date = df.iloc[0, :]['current_date']
end_date = df.iloc[1, :]['current_date']
# If the start_date is valid, convert it into a datetime
if not pd.isnull(start_date):
start_date = datetime64_to_datetime(start_date)
else:
# No start_date, use 90 days after the prior_date
start_date = datetime64_to_datetime(df.iloc[0, :]['prior_date']) + dt.timedelta(days=90)
# If the end_date is valid, convert it into a datetime
if not pd.isnull(end_date):
end_date = datetime64_to_datetime(end_date)
else:
# No end date, use 90 days after the prior_date
end_date = datetime64_to_datetime(df.iloc[1, :]['prior_date']) + dt.timedelta(days=90)
days_between = (end_date - start_date).days
gallons_per_day = difference_in_gallons / days_between
df_gpd.loc[index, 'GPD'] = gallons_per_day
print("Done!\n")
print("Sorting table by street name, then by house number")
df_gpd = df_gpd.sort_values(by=['address_street_name', 'address_street_number'])
print("Done!\n")
print("Creating table of street GPDs...")
# Create a dataframe for each street
street_gpd_base_dict = {'street': [], 'GPD': []}
street_gpd = pd.DataFrame(street_gpd_base_dict)
for street in street_names:
house_data = df_gpd[df_gpd['address_street_name'] == street]
gpd = house_data['GPD'].sum()
street_gpd.loc[len(street_gpd)] = [street, gpd]
street_gpd = street_gpd.sort_values(by=['street'])
print("Done!\n")
elapsed_time = time.time() - start_time
# Round elapsed time to 2 decimal places
print("Elapsed time: {0} seconds".format(round(elapsed_time * 100) / 100))
# Returns the number of houses with more than min_gpd gpd
def count_by_min_gpd(min_gpd):
temp_df = df_gpd[df_gpd['GPD'] > min_gpd]
return len(temp_df.index)
print('\n{0} households have an average GPD greater than 300'.format(count_by_min_gpd(300)))
print('{0} households have an average GPD greater than 500'.format(count_by_min_gpd(500)))
print('{0} households have an average GPD greater than 1000\n'.format(count_by_min_gpd(1000)))
print("Saving GPD data to 'household_gpd.csv'...")
df_gpd.to_csv('household_gpd.csv', index=False)
print('Done!\n')
print("Saving street GPD data to 'streets.csv'...")
street_gpd.to_csv('streets.csv', index=False)
print('Done!\n')
print("Saving all GPD data to 'gpd.xlsx'...")
# Write to Excel sheet
with pd.ExcelWriter('gpd.xlsx') as writer:
df_gpd.to_excel(writer, index=False, sheet_name='Household GPD')
street_gpd.to_excel(writer, index=False, sheet_name='Street GPD')
print("Done!")
Building table of average gallons used per day (average GPD)...
Done!
Calculating all average GPDs...
Done!
Sorting table by street name, then by house number
Done!
Creating table of street GPDs...
Done!
Elapsed time: 86.3 seconds
403 households have an average GPD greater than 300
94 households have an average GPD greater than 500
14 households have an average GPD greater than 1000
Saving GPD data to 'household_gpd.csv'...
Done!
Saving street GPD data to 'streets.csv'...
Done!
Saving all GPD data to 'gpd.xlsx'...
Done!
import os
from distutils.core import setup
from Cython.Build import cythonize
setup(ext_modules=cythonize(os.path.join('SQLite', 'main.py')))
I run the code by running
python3.6 cython-setup.py build_ext --inplace
import SQLite.main